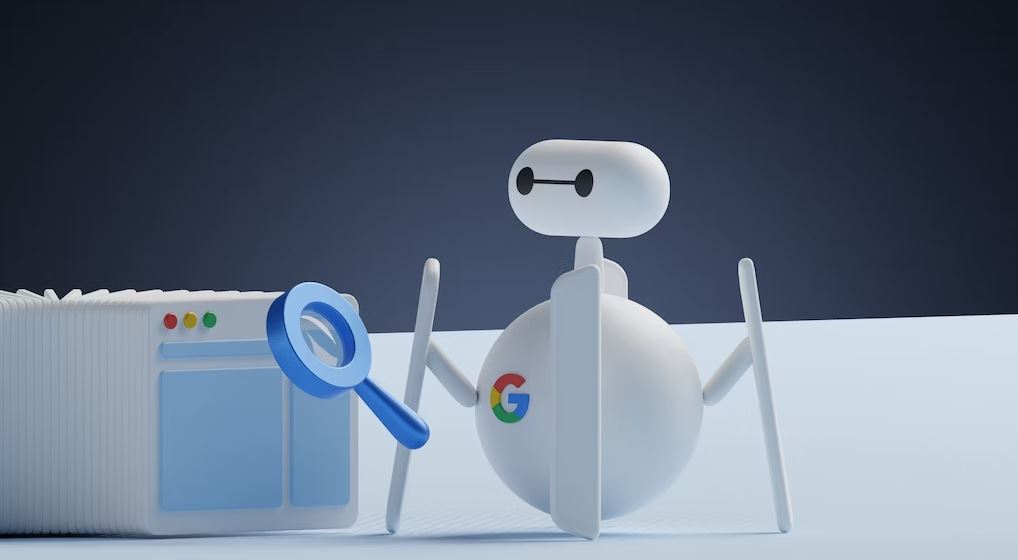
In today’s digital age, businesses and organizations are inundated with vast amounts of data. From customer information to transaction records, this wealth of data holds immense potential. However, the true value lies in leveraging this data to make informed decisions and optimize processes. Optimization algorithms, coupled with advanced analytics techniques, have emerged as powerful tools for harnessing the power of data and driving better decision making in various domains.
One of the key aspects of optimization in the digital age is the concept of prescriptive analytics. Unlike descriptive and predictive analytics, which focus on understanding past events and predicting future outcomes, prescriptive analytics aims to provide recommendations on the best course of action to optimize desired outcomes. By analyzing historical data, identifying patterns, and considering various constraints, optimization algorithms can generate actionable insights that enable organizations to make informed decisions.
Optimization in the Decision Making for Better Idea
A prominent area where optimization algorithms have made a significant impact is pricing optimization. Pricing decisions are critical for businesses as they directly impact profitability. Optimization algorithms can analyze historical sales data, market conditions, and customer behavior to determine the optimal pricing strategy. By considering factors like demand elasticity, competitor pricing, and cost structures, these algorithms can recommend optimal price points to maximize revenue or profit margins. Such optimization-driven pricing strategies have been successfully implemented in industries ranging from e-commerce to hospitality, revolutionizing revenue management practices.
Another area where optimization plays a vital role is demand forecasting and inventory management. With optimization algorithms, organizations can analyze historical sales data, market trends, and external factors like seasonality or promotions to accurately forecast future demand. This enables businesses to optimize inventory levels, minimize stockouts, and reduce carrying costs. By aligning supply with demand, organizations can streamline operations, enhance customer satisfaction, and improve overall profitability. Optimization-driven demand forecasting and inventory management have proven particularly valuable in industries like retail, manufacturing, and logistics.
Optimization algorithms are also instrumental in improving operational efficiency and resource allocation. In sectors such as transportation and logistics, organizations face the challenge of optimizing routes, schedules, and vehicle utilization. By incorporating data on variables like delivery locations, traffic conditions, and vehicle capacities, optimization algorithms can optimize routes and schedules to minimize costs, reduce delivery time, and improve resource utilization. The result is enhanced operational efficiency, reduced fuel consumption, and improved customer service.
The digital age has witnessed the emergence of machine learning-based optimization, which combines the power of optimization algorithms with the capabilities of machine learning. Machine learning algorithms can learn patterns and make predictions from large datasets, while optimization algorithms can refine these predictions and generate optimized solutions. This synergy has opened up new possibilities in fields like healthcare, energy management, and predictive maintenance.
In healthcare, for example, optimization algorithms can optimize patient scheduling, resource allocation, and treatment planning. By considering factors like patient priorities, available resources, and scheduling constraints, these algorithms can help hospitals and clinics allocate resources effectively, reduce waiting times, and improve patient outcomes. In the energy sector, optimization algorithms can optimize power generation and distribution, taking into account factors like demand patterns, renewable energy availability, and cost considerations. This enables utilities to optimize energy production, minimize costs, and reduce environmental impact.
However, while optimization in the digital age offers immense potential, it also comes with challenges. Data quality, data privacy, and computational complexity are among the key considerations organizations must address when implementing optimization algorithms. Ensuring data accuracy and reliability is crucial for generating meaningful insights and optimal solutions. Additionally, organizations need to adhere to data privacy regulations to protect sensitive information while still utilizing data effectively.
Optimization algorithms have become invaluable tools for organizations seeking to leverage data for better decision making in the digital age. By combining advanced analytics techniques with optimization methodologies, businesses can unlock efficiency, optimize processes, and drive improved outcomes.
Optimization in the Digital Age: Leveraging Data for Better Decision Making:
- Introduction to Optimization in the Digital Age
- The increasing availability of data and advancements in computing power have led to the emergence of optimization algorithms in decision making.
- Optimization algorithms aim to provide actionable insights and recommendations for organizations to achieve desired outcomes.
- Prescriptive analytics plays a key role in optimization, offering the best course of action based on data analysis and constraints.
- Pricing Optimization: Maximizing Revenue and Profitability
- Optimization algorithms analyze historical data, market conditions, and customer behavior to determine optimal pricing strategies.
- By considering demand elasticity, competitor pricing, and cost structures, these algorithms recommend price points that maximize revenue or profit margins.
- Pricing optimization has transformed revenue management practices in industries such as e-commerce and hospitality.
- Demand Forecasting and Inventory Management: Aligning Supply and Demand
- Optimization algorithms analyze historical sales data, market trends, and external factors to forecast future demand accurately.
- Organizations can optimize inventory levels, minimize stockouts, and reduce carrying costs by aligning supply with demand.
- Industries like retail, manufacturing, and logistics benefit from optimization-driven demand forecasting and inventory management.
- Operational Efficiency and Resource Allocation: Streamlining Operations
- Optimization algorithms optimize routes, schedules, and resource utilization in sectors such as transportation and logistics.
- By incorporating data on delivery locations, traffic conditions, and vehicle capacities, algorithms minimize costs, reduce delivery time, and improve efficiency.
- Improved operational efficiency leads to reduced fuel consumption and enhanced customer service.
- Machine Learning-based Optimization: Combining Analytics and Optimization
- Machine learning algorithms learn patterns and make predictions from large datasets, while optimization algorithms refine these predictions and generate optimized solutions.
- Healthcare, energy management, and predictive maintenance are areas benefiting from machine learning-based optimization.
- Optimization algorithms optimize patient scheduling, resource allocation, power generation, and distribution, resulting in improved outcomes and reduced costs.
- Challenges and Considerations in Optimization
- Data quality and accuracy are crucial for generating meaningful insights and optimal solutions.
- Organizations must adhere to data privacy regulations to protect sensitive information while utilizing data effectively.
- Computational complexity and scalability are challenges that need to be addressed when implementing optimization algorithms.
Conclusion: Optimization algorithms, in conjunction with data analytics, have become essential tools for organizations to make data-driven decisions in the digital age. From pricing optimization to demand forecasting and operational efficiency, these algorithms help businesses achieve better outcomes, reduce costs, and enhance customer satisfaction. Despite challenges, organizations can harness the power of optimization to leverage data effectively and gain a competitive advantage in today’s dynamic business landscape.